Google’s Multitask Unified Model (MUM) represents a significant advancement in search technology, operating 1,000 times more powerfully than its BERT predecessor. This AI-driven system processes 75 languages simultaneously while understanding multiple content formats including text, images, and video. MUM’s sophisticated blueprint enables deeper comprehension of user intent and complex search queries, fundamentally shifting SEO strategies from traditional keyword refinement. For digital marketers and content creators, this necessitates a more subtle approach focusing on holistic, situationally relevant content that addresses user intent across multiple formats. The evolution of MUM signals revolutionary changes in how businesses enhance their digital presence.
Learn More
- MUM processes content across 75 languages and multiple formats, requiring SEO strategies that optimize for global, multilingual audiences.
- Content quality and contextual relevance are prioritized over traditional keyword optimization in MUM-focused SEO approaches.
- MUM’s advanced natural language understanding capabilities demand SEO content that addresses user intent rather than exact keyword matches.
- The algorithm’s multimodal processing abilities necessitate optimization of various content formats, including text, images, and video.
- SEO strategies must evolve beyond single-language keyword targeting due to MUM’s cross-lingual content analysis capabilities.
Understanding Google MUM
Search engines underwent a revolutionary metamorphosis with the introduction of Google MUM (Multitask Unified Model), representing a significant leap in artificial intelligence-powered search technology.
This sophisticated planner leverages natural language processing and deep learning capabilities to comprehend and process search queries with unparalleled sophistication, moving well beyond traditional keyword-based search mechanisms. MUM utilizes the T5 text-to-text framework to unprecedentedly process and understand information. The system’s advanced capabilities allow it to process 75 different languages simultaneously. Effective implementation requires thorough technical optimization to ensure maximum visibility in search results.
MUM’s blueprint demonstrates extraordinary versatility in simultaneously processing multiple languages and data formats. Unlike its predecessors, MUM operates with a holistic understanding of user intent, background, and content relevance across diverse media formats, including text, images, videos, and audio content.
This multimodal approach enables the algorithm to deliver more subtle and accurate search results.
The technology exhibits superior processing capabilities, operating at 1000 times the power of BERT, its predecessor. This amplified processing power allows MUM to analyze complex queries and deliver results demonstrating a deeper understanding of user needs.
The algorithm’s ability to break down language barriers represents a significant advancement in search technology. It provides relevant results regardless of the query’s original language.
As search technology evolves, MUM’s implementation signals a fundamental transformation in how search engines interpret and fulfill user queries. Its sophisticated understanding of world knowledge and its ability to process multiple tasks simultaneously create a more intelligent and efficient search experience.
This advancement necessitates fundamentally reconsidering traditional SEO approaches, emphasizing the importance of exhaustive, high-quality content that aligns with user intent rather than solely focusing on keyword optimization.
Key Features and Capabilities
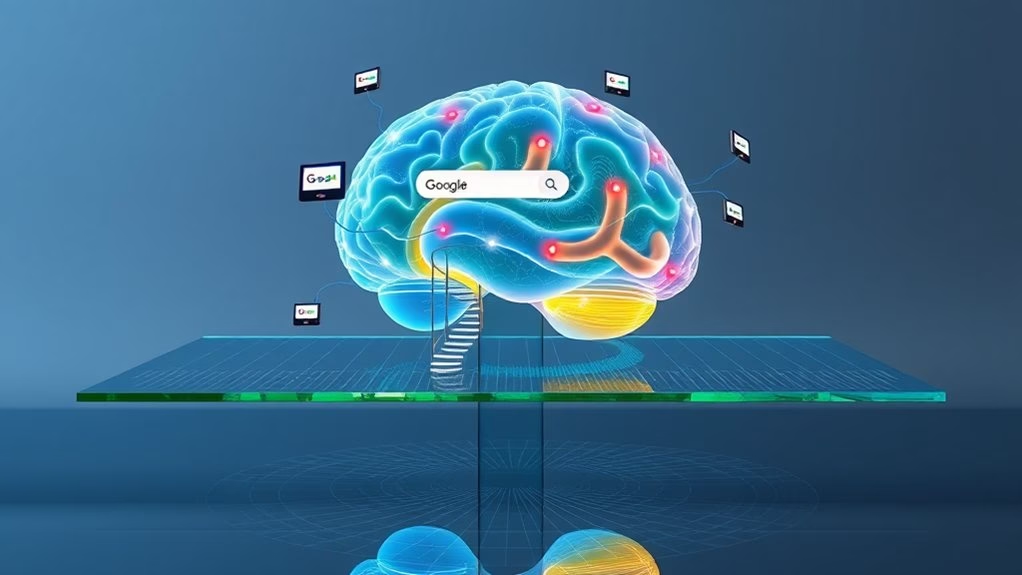
Google MUM’s revolutionary blueprint defines three core capabilities: multimodal processing, multitasking abilities, and advanced language understanding.
The system’s multimodal processing blueprint enables seamless analysis of diverse content formats, including text, images, audio, and video. Through integration with Google Lens, MUM processes visual queries while its specialized text understanding (TUMT) and text merging (TMT) modules generate situationally relevant responses. Built on a transformer architecture, this system delivers unprecedented processing capabilities. This advanced system is dramatically faster than previous search technologies.
The multitasking capabilities represent a significant advancement in search technology. MUM’s blueprint facilitates concurrent execution of multiple complex tasks through tightly coupled modules that share weights and activations. This innovative approach helps reduce search friction through comprehensive information retrieval across platforms.
This design enables simultaneous keyword grouping, multilingual analysis, and cross-format content searching. The system’s end-to-end machine learning approach makes certain efficient handling of complex multimodal tasks, particularly in supporting Google Assistant and Google Search functionalities.
MUM’s language and content understanding capabilities extend across 75 languages, marking a substantial improvement in multilingual search processing. The system’s ability to transfer knowledge between languages enables holistic analysis of global content sources.
This multilingual blueprint allows MUM to extract observations from content in various languages, combine information, and deliver relevant results regardless of the source language. By simultaneously processing queries in multiple formats and understanding cross-language relationships, MUM represents a significant evolution in search technology, offering more layered and holistic search capabilities than previous generations of search algorithms.
MUM vs. BERT
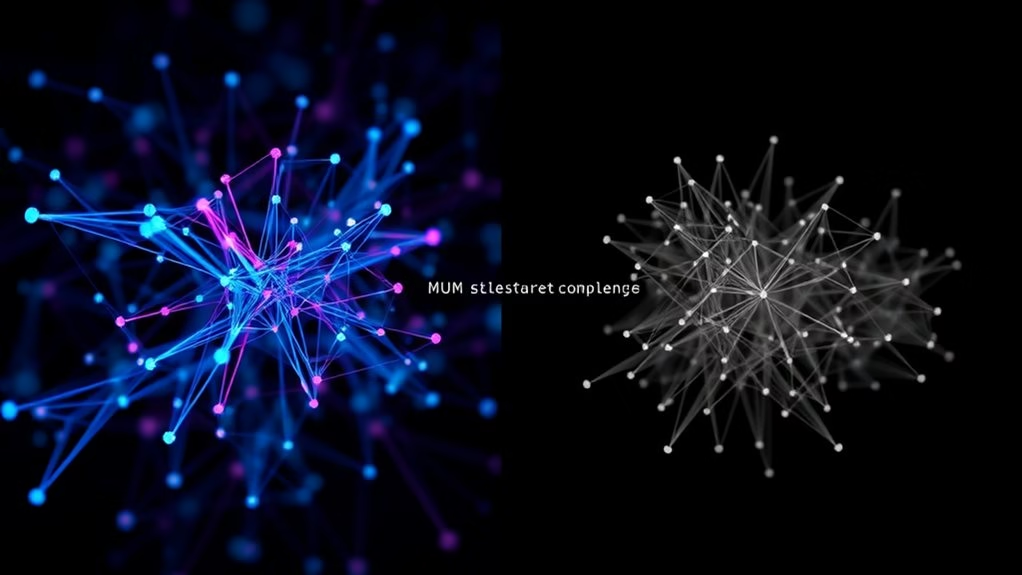
Google MUM’s processing capabilities significantly advance over BERT, demonstrating 1,000 times more power in handling complex search queries. The system employs a T5 text-to-text framework for enhanced processing.
While BERT transformed natural language processing with its situational understanding, MUM expands this foundation by simultaneously processing queries across 75 languages.
MUM’s augmented language interpretation enables it to grasp subtle meanings and provide holistic answers with fewer searches, marking a substantial evolution in search technology’s ability to understand and respond to user intent. MUM utilizes transformer architecture to achieve its sophisticated language processing capabilities. Its innovative design accepts multiple formats, including text, images, and videos, for comprehensive search results.
Processing Power Comparison
A monumental leap in processing capabilities separates MUM from its predecessor, BERT. MUM demonstrates 1,000 times more computational power and the ability to handle multiple tasks simultaneously.
This improved processing capacity enables MUM to analyze complex queries across multiple languages, data formats, and media types in a single operation, significantly reducing the computational burden on servers. The system achieves remarkable resource savings of up to 80% during search operations.
MUM supports understanding 75 different languages simultaneously, making it a truly global search solution.
MUM’s design, built on the T5 text-to-text blueprint and incorporating HyperGrid Transformers, facilitates efficient multi-task learning and processing.
Unlike BERT’s sequential, single-language processing approach, MUM can seamlessly interpret text, images, and videos concurrently, leveraging its advanced Transfer Learning capabilities to understand setting across diverse data sources.
Implementing MoSE-like technologies further boosts MUM’s efficiency by predicting and incorporating sequential search behaviors.
Combined with MUM’s parallel processing abilities, this predictive capability dramatically reduces the number of complex queries that must be searched.
The result is a more optimized search experience that requires fewer computing resources while providing faster, more inclusive results across multiple languages and formats.
Language Understanding Capabilities
With MUM’s introduction, language understanding capabilities have evolved dramatically, significantly departing from BERT’s more limited linguistic processing abilities. With training across 75 languages and advanced multilingual understanding, MUM demonstrates superior situational perception and simultaneous language processing capabilities. The system’s text-to-text framework enables more sophisticated processing of search queries. The platform leverages expert-level insights to provide deeper understanding across numerous subject areas.
Feature | MUM | BERT |
---|---|---|
Language Coverage | 75 languages simultaneously | Single language processing |
Query Processing | Complex, multi-intent queries | Basic semantic understanding |
Content Format | Text, images, audio | Primarily text-based |
Translation Ability | Cross-language information synthesis | Limited translation capacity |
Situational Analysis | Advanced situational understanding | Basic situational processing |
MUM’s sophisticated language processing extends beyond traditional text analysis, incorporating multimodal capabilities that enable it to interpret and correlate information across different content formats. This advancement particularly impacts multilingual SEO strategies, as MUM can effectively bridge language barriers by simultaneously processing and translating content across multiple languages. The system’s refined interpretive understanding allows it to grasp delicate query intent and deliver more relevant results, representing a significant evolution in search technology’s ability to comprehend and respond to user queries across linguistic parameters. Being 1,000 times more powerful than its predecessor, MUM represents a quantum leap in natural language processing capabilities.
Impact on Search Results
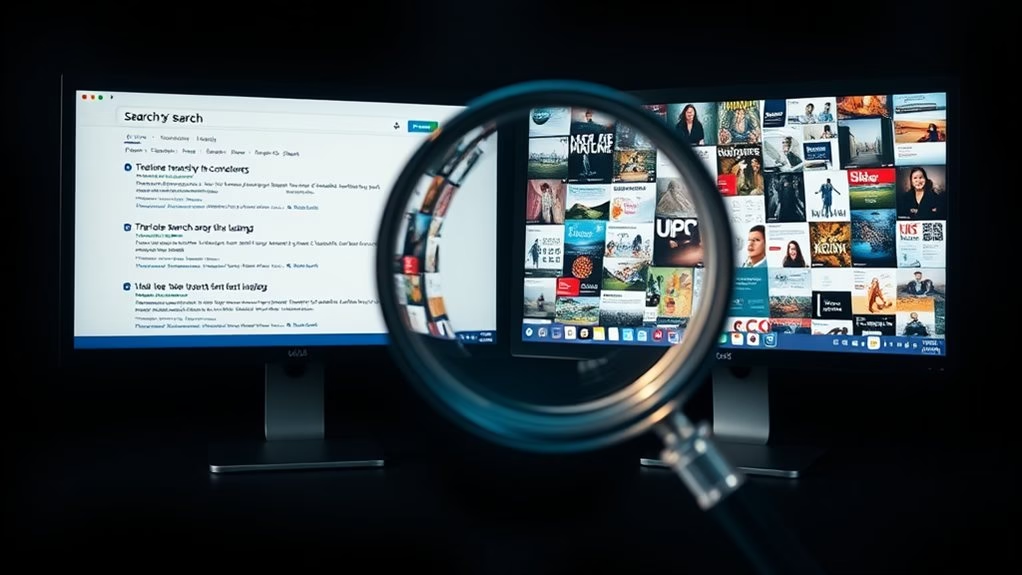
Modern search engines undergo a revolutionary metamorphosis with the introduction of MUM, which fundamentally reshapes how search results are delivered and interpreted.
This technological advancement significantly boosts result relevancy through sophisticated multimodal understanding and holistic situational analysis, enabling faster and more accurate information retrieval across global markets. The system’s continuous machine learning ensures search results become increasingly refined. MUM demonstrated its capabilities by processing 800 vaccine variations during the COVID-19 pandemic. The system’s semantic search capabilities significantly shift from traditional keyword-matching approaches.
MUM’s impact on search results manifests in several critical ways. The system’s ability to process multiple content types simultaneously allows for more subtle result delivery, incorporating text, images, and video content into a cohesive search experience.
This multimodal processing capability, combined with heightened situational understanding, enables MUM to deliver search results that more precisely match user intent, reducing the necessity for multiple search iterations.
The system’s global accessibility features significantly advance cross-linguistic search functionality. By analyzing content across multiple languages simultaneously, MUM breaks down traditional language barriers, ensuring users receive relevant results regardless of their geographic location or preferred language.
This capability particularly benefits international SEO strategies and content distribution efforts.
Furthermore, MUM’s emphasis on content quality and relevance affects result rankings. The algorithm’s sophisticated understanding of user intent means high-quality, situationally pertinent content receives greater visibility in search results.
This advancement particularly benefits content that addresses specific user needs through holistic, well-structured information. It also considers geographical factors and server locations in its ranking determinations.
SEO Strategy Adaptations
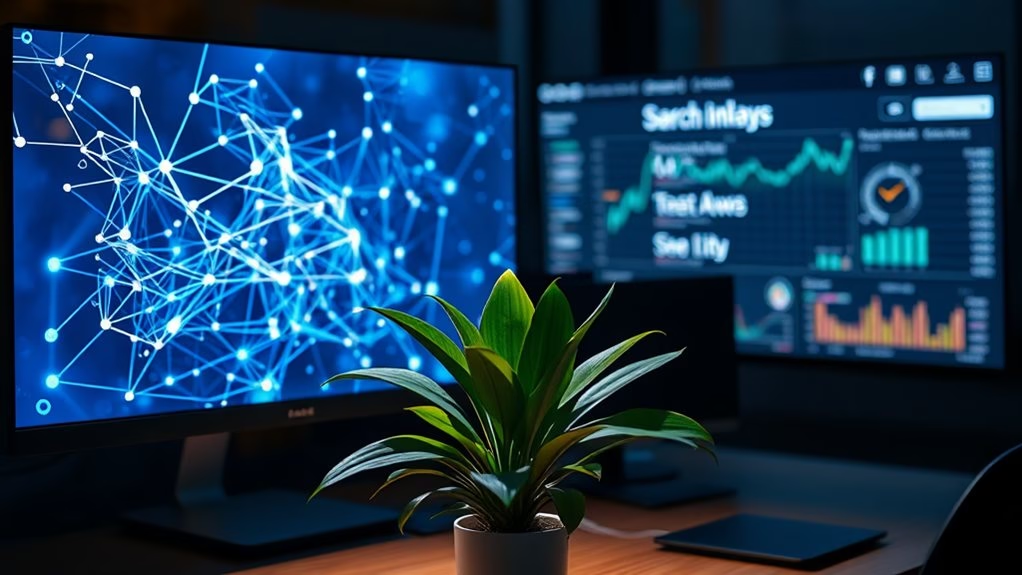
Successful SEO strategies must undergo significant metamorphosis to align with Google MUM’s advanced capabilities and shifting terrain. Organizations must pivot from traditional keyword-centric approaches to exhaustive content strategies that address user intent across multiple modalities and languages.
Strategy Component | Required Modification |
---|---|
Content Creation | Shift to profound, exhaustive content that addresses broader background and multiple aspects of user queries |
Keyword Approach | Focus on semantic relationships and user intent rather than isolated keyword density |
Technical Elements | Fine-tune for multimodal content delivery and guarantee sturdy mobile performance |
User Experience | Prioritize proficiency, authority, and trust while developing new engagement metrics |
The evolution of content optimization demands an all-encompassing approach, emphasizing quality over quantity. Content must now demonstrate an exhaustive understanding of topics, incorporating various media formats while maintaining situational relevance. This includes developing detailed, authoritative content that addresses user needs rather than creating multiple shallow pieces. The system’s ability to process content across 75 languages enables unprecedented global content reach and engagement opportunities.
Technical considerations have expanded beyond traditional SEO parameters. Websites must now support diverse content types, including images, videos, and audio while maintaining optimal performance metrics. This multimodal approach requires careful attention to site design and loading speeds across devices.
Success metrics are also metamorphosing, with traditional SEO KPIs giving way to more subtle measurements of user engagement and content effectiveness. Organizations must develop new models for measuring content performance that align with MUM’s sophisticated understanding of user intent and cross-lingual capabilities.
Future of Search Technology
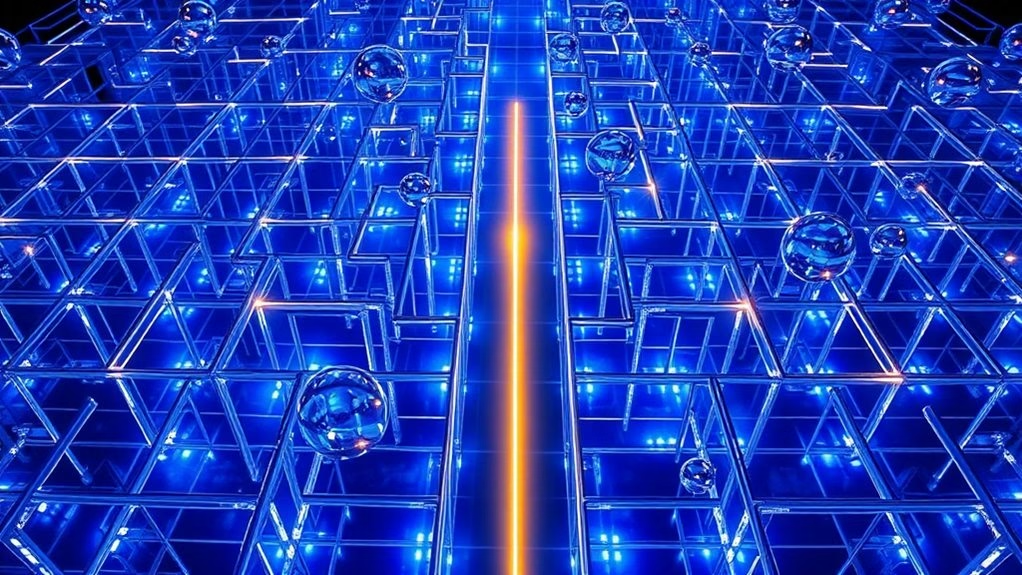
The rapid evolution of search technology, driven by Google MUM’s sophisticated capabilities, signals a revolutionary shift in how users interact with digital information.
Through advanced AI-driven search mechanisms and unified modeling approaches, search engines are prepared to deliver increasingly subtle and situationally relevant results across multiple languages and data formats.
Integrating advanced natural language processing capabilities and multimodal analysis suggests a future where search engines will comprehend and process complex queries with unparalleled accuracy.
The combination of text, image, video, and audio analysis within a single consolidated structure represents a significant leap forward in search technology’s ability to understand and serve user intent across diverse media formats.
As search technology continues to evolve, expanding knowledge graphs and semantic databases will enable more sophisticated situational understanding.
This advancement and continuous algorithm updates and AI integration point toward a future where search results become increasingly tailored and scenario-aware.
The development of text-to-video AI tools and adaptive layouts further indicates a shift toward more visually oriented search experiences.
The path of search technology suggests a future characterized by semantic search evolution and advanced multilingual capabilities, supporting seamless information access across 75 languages.
This progression, supported by improved efficiency in machine learning models and expanded data source integration, will likely reshape how users uncover and interact with information online.
The continuous improvement of search algorithms and increased reliance on NLP will further amplify the precision and relevance of search results.
Frequently Asked Questions
How Long Does It Take for MUM to Analyze a Website Fully?
While exact analysis times aren’t publicly disclosed, MUM processes websites near-instantaneously through its advanced AI capabilities, analyzing multimodal content simultaneously. Processing speed varies based on site complexity and content volume.
Can Website Owners Opt Out of Mum’s Analysis of Their Content?
There is no direct opt-out mechanism for website owners to exclude their content from MUM’s analysis. Content analysis is integral to how the system processes and understands web information.
Does MUM Impact Local Search Results Differently Than Global Searches?
Local searches experience distinct impacts through amplified geographic specificity, stronger cultural background interpretation, and heightened local business visibility while potentially affecting click-through rates differently than broader global search queries.
What Are the Minimum Technical Requirements for Mum-Optimized Content?
Essential technical requirements for MUM-optimized content include mobile responsiveness, structured data markup, multimodal elements, semantic HTML5, fast loading speeds, accessible design features, and content available in multiple languages with proper hreflang implementation.
How Frequently Does Google Update Mum’s Learning Models and Algorithms?
Since its 2021 launch, MUM hasn’t received major public updates. While continuous improvements occur through Search Quality Rater Guidelines and human feedback, Google maintains discretion about specific update frequencies for their learning models.
Conclusion
Google’s Multitask Unified Model (MUM) represents a revolutionary approach to search technology, fundamentally reshaping how content is understood and ranked. As this AI-driven system evolves, SEO strategies must adjust to emphasize holistic topic coverage, multilingual optimization, and multimodal content integration. Organizations that align their digital presence with MUM’s capabilities while maintaining core SEO principles will be better positioned to achieve visibility in this emerging epoch of semantic search.