Knowledge graphs significantly boost search engine optimization through structured data relationships and semantic connections. These graph-based systems improve organic traffic by up to 35% while strengthening click-through rates and search result visibility. Technical implementation requires holistic Schema.org markup, specialized graph databases, and strategic entity relationship optimization. Modern SEO benefits from knowledge graphs through improved featured snippets, knowledge panels, and zero-click search functionalities. The integration of knowledge graphs with natural language processing creates more accurate search matching and advanced user experiences. Understanding the full scope of knowledge graph implementation reveals powerful opportunities for digital presence optimization.
Learn More
- Knowledge graphs enhance SEO performance by increasing organic traffic up to 35% and improving click-through rates on search results.
- Implementing Schema.org markup helps search engines understand website content and establish entity relationships for better rankings.
- Knowledge graphs enable featured snippets and knowledge panels, providing immediate answers to user queries in search results.
- Strategic implementation across multiple platforms strengthens digital presence and improves semantic search understanding.
- Knowledge graphs connect related entities and concepts, supporting zero-click search functionalities and enhanced user search experience.
Understanding Knowledge Graphs
Knowledge graphs represent a sophisticated approach to organizing and interconnecting data through a graph-structured model that captures both entities and their relationships.
This structured representation consists of nodes that represent entities and edges that define the relationships between these entities, creating an all-encompassing network of interconnected information. At its core, a knowledge graph leverages an ontology to encode semantic meaning, enabling both human users and machines to interpret and process the data effectively. These systems excel at data science applications, supporting advanced analytics and artificial intelligence solutions. The technology's origins date back to 1972 when Austrian linguist Schneider first introduced the concept. The native relationship tracking enables seamless navigation between connected data points.
The adaptive nature of knowledge graphs allows them to evolve continuously, reflecting changes in their respective domains while incorporating new information as it becomes available.
These graphs can be constructed through various methods, including manual creation, machine learning from unstructured data, or integration of existing knowledge bases. The underlying schema plays a pivotal role in organizing and validating the data, ensuring consistency and accuracy across the entire graph structure.
Knowledge graphs exist in several forms, ranging from open-source implementations like DBpedia and Wikidata to enterprise-specific solutions such as Google's Knowledge Graph.
Specialized versions are implemented across various sectors, including healthcare and financial services, while personal knowledge graphs are increasingly employed in individual knowledge management systems.
The implementation of knowledge graphs typically relies on specialized graph databases like Neo4j and GraphDB, which provide the necessary infrastructure for storing and managing complex graph-structured data.
This technological foundation enables key benefits such as situational understanding, improved reasoning capabilities, and efficient data integration, making knowledge graphs particularly significant for applications in search engines, recommendation systems, and artificial intelligence platforms.
Impact on Modern SEO

The integration of knowledge graphs into search engine algorithms has fundamentally reshaped modern SEO practices, with data showing significant impacts on organic traffic and user engagement metrics.
E-commerce platforms have reported up to 35% increases in click-through rates from search engine results pages, with a consistent 20% uplift in organic traffic following knowledge graph implementation. In healthcare sectors, implementation has led to a 25% boost in organic bookings for medical services.
The impact extends beyond mere traffic metrics, influencing search engine rankings through upgraded information access and improved matching of user search intent. Semantic understanding has revolutionized how search engines interpret and deliver results. Since its launch in 2012, Google's Knowledge Graph has continuously evolved to enhance semantic search capabilities.
While knowledge graphs can reduce website traffic through zero-click searches, they simultaneously provide authority signals that influence SEO rankings. Organizations optimizing their content with structured data through Schema.org markup demonstrate improved search visibility and ranking potential.
User experience metrics have become increasingly central to SEO success, with knowledge graphs conveying immediate, accurate information through rich snippets and knowledge panels.
This metamorphosis has necessitated a shift in SEO strategy, focusing on holistic content optimization that aligns with semantic search principles. Data indicates that properly structured content amplifies visibility through knowledge cards and increases the likelihood of integration in knowledge graph results.
To maximize SEO effectiveness in the knowledge graph era, businesses must modify their strategies to focus on niche topics where knowledge graph dominance is limited.
This approach, combined with strong structured data implementation and content accuracy optimization, creates an all-encompassing SEO blueprint that capitalizes on knowledge graphs' benefits while mitigating potential traffic losses from zero-click searches.
Leveraging Knowledge Graphs
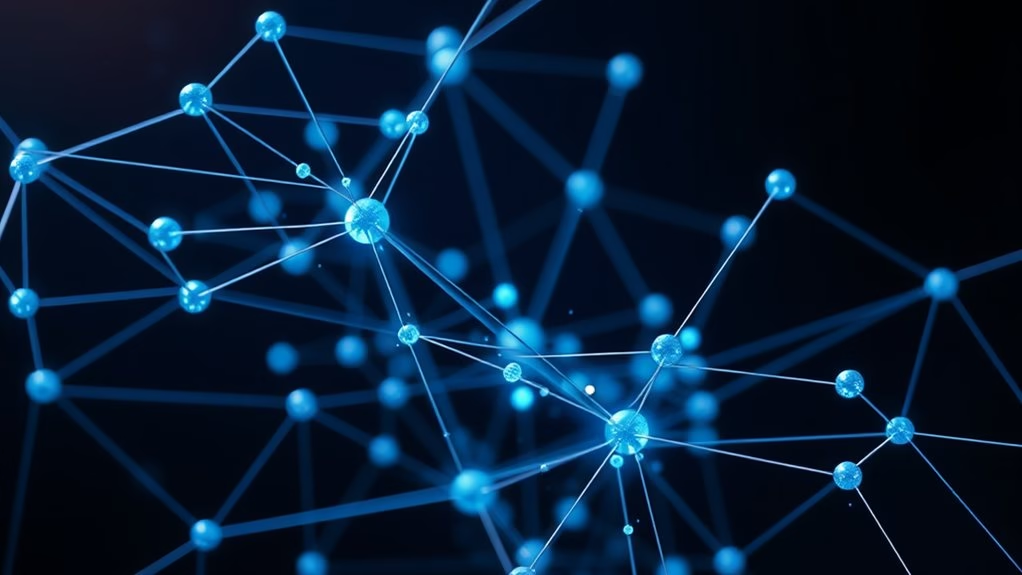
Successfully leveraging knowledge graphs for SEO requires a multi-faceted approach centered on structured data implementation and entity optimization.
Organizations must focus on implementing Schema.org markup while establishing authoritative presence across multiple digital platforms to amplify their visibility within Google's Knowledge Graph ecosystem. This comprehensive database has been powering search features since 2012. Graph-based integration enables seamless connection between related entities and concepts. The database helps users discover quick factual answers without clicking through to websites.
Strategic optimization involves scrupulous attention to entity relationships and semantic search integration.
By incorporating structured data markup for key entities such as Person, Organization, and LocalBusiness, websites can provide search engines with precise setting about their content.
This technical foundation must align with holistic E-A-T criteria implementation to strengthen domain authority and trustworthiness.
- Implement Schema.org markup strategically across all relevant entity types
- Verify and claim Knowledge Panel ownership through Google's verification process
- Establish authoritative presence on platforms like Wikipedia, LinkedIn, and industry databases
- Develop internal knowledge graphs to bolster entity relationships and content connectivity
The integration of social media optimization and entity recognition plays pivotal role in expanding digital footprint and authority.
Organizations must modify to zero-click search trends by optimizing their content to appear in featured snippets and knowledge panels.
This approach requires careful consideration of user intent and query patterns, ensuring that structured data implementations align with natural language processing requirements.
Through systematic implementation of these strategies, organizations can amplify their visibility in search results while future-proofing their SEO efforts against algorithm updates.
Search Engine Applications
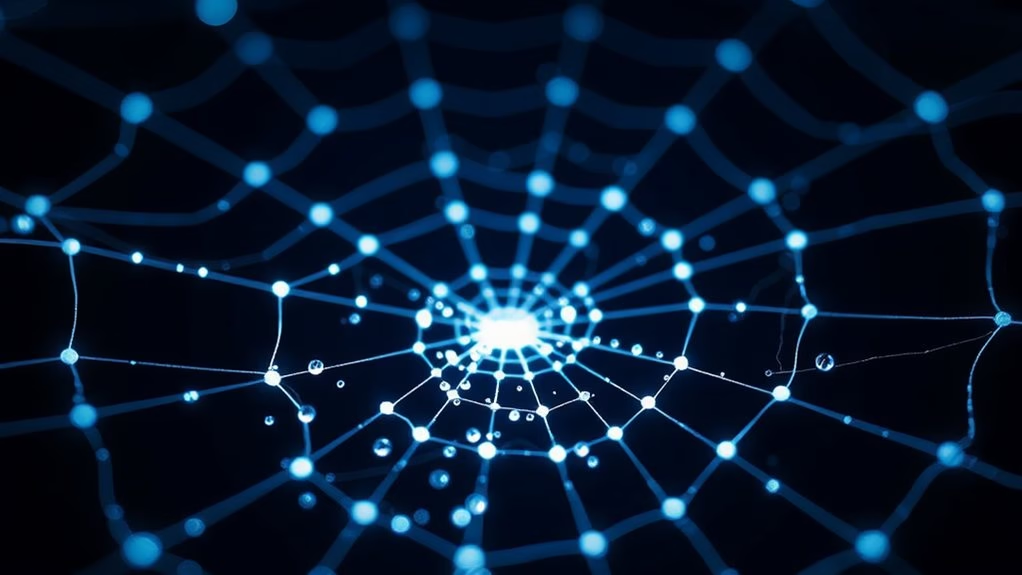
Modern search engines extensively utilize knowledge graphs to revolutionize information retrieval and query processing capabilities. Industry leaders like Google, Microsoft's Bing, and Facebook have implemented sophisticated knowledge graph systems that amplify their search functionalities through structured data relationships and semantic understanding. The implementation of RDF triple formats enables standardized information representation across platforms. Advanced entity recognition algorithms enhance the accuracy of search results through systematic identification of key data points.
Google's Knowledge Graph stands as a prime example, providing detailed entity information directly in search results, while Microsoft's Satori knowledge graph powers Bing's ability to process complex queries through structured information presentation. Search result enrichment enables comprehensive contextual details to be displayed alongside standard results. Facebook's Graph Search differentiates itself by incorporating user relationships and preferences, creating tailored search experiences based on social connections.
These implementations significantly impact search performance through multiple mechanisms. Knowledge graphs enable semantic search capabilities that better interpret user intent, facilitating more accurate result transmission.
By integrating diverse data sources and establishing relationships between entities, these systems can handle intricate queries that traditional keyword-based search would struggle to process effectively.
The technical design of search engine knowledge graphs emphasizes data accuracy and situational awareness. Through structured data implementation and schema markup, search engines can better understand and present information in rich formats.
This systematic approach to data organization allows for efficient processing of complex relationships between entities, enabling search engines to provide direct answers and related information within search results.
The integration of knowledge graphs has fundamentally altered how search engines interpret and respond to user queries, establishing a more sophisticated and scenario-aware search model that extends beyond simple keyword matching.
Future Trends and Challenges

Despite significant advances in knowledge graph implementation, emerging challenges and future developments signal major shifts in SEO practices and search technology terrains.
Data complexity presents substantial technical obstacles, particularly in knowledge acquisition, integration, and embedding processes. Current technologies demonstrate notable limitations in effectively consolidating information from diverse sources, while scalability and data quality issues impede optimal knowledge graph implement. Virtual assistants and AI-powered chatbots are reshaping how users interact with websites and search for information. The evolution from a keyword-based approach to contextual search has fundamentally transformed how search engines process information. The emphasis on Core Web Vitals continues to shape technical optimization requirements for better search performance.
The integration of AI and machine learning in search engines has fundamentally altered the search ecosystem. Google's Helpful Content Update, combined with sophisticated AI systems, emphasizes the critical need for high-quality, proficiency-driven content that aligns with E.A.T guidelines.
These developments necessitate a strategic pivot in SEO approaches, focusing on extensive content knowledge graphs and situational relevance beyond traditional keyword optimization.
Key future trends that will reshape SEO practices include:
- Rise of AI-powered search systems like SearchGPT, challenging conventional search engine dominance
- Emergence of customized knowledge graphs, emphasizing brand SERP optimization
- Growing importance of structured data and content knowledge graphs in SEO strategies
- Evolution toward more interactive and tailored search experiences
The fragmentation of the search ecosystem requires flexible SEO strategies that accommodate multiple platforms and AI-driven interfaces. As search engines become increasingly sophisticated in understanding user intent, the effective implementation of knowledge graphs becomes paramount for maintaining online visibility and authority.
This technological evolution demands a more subtle approach to content creation and optimization, focusing on extensive knowledge integration and situational relevance.
Case Studies in Action

Real-world implementations of Knowledge Graphs demonstrate significant SEO performance improvements across diverse industries, with companies reporting organic traffic increases ranging from 26.8% to 198%. Effective knowledge graph optimization requires semantic networks that map relationships between concepts and entities.
Analysis of multiple case studies reveals consistent patterns of success, including amplified rich snippet visibility, improved SERP rankings, and substantial growth in click-through rates, exemplified by an e-commerce giant's 35% CTR increase. Glasses.com's content optimization delivered impressive results, achieving a 27.78% increase in organic clicks.
The implementation of structured data markup, particularly Schema.org, combined with strategic Knowledge Graph optimization, has led to documented successes such as Express Legal Funding's 4.7x organic traffic growth and SalzburgerLand Tourismus's 92.65% surge in organic search users.
Real-World Success Stories
Leading organizations implementing Knowledge Graphs in their SEO strategies have documented extraordinary success metrics across multiple performance indicators.
Notable achievements include a 35% increase in click-through rates from search engine results pages and a 20% uplift in organic traffic through amplified data structuring. These improvements directly correlate with increased visibility in rich snippets and voice search results. The integration of Schema markup language has been crucial for these improvements. Entity relationships enable Google to deliver more contextually relevant search results.
Strategic implementation of Knowledge Graphs has yielded exceptional results across various sectors, particularly in e-commerce and retail. Industry data reveals several key performance metrics:
- 70% of key products achieving first-page Google rankings for retail clients
- 45% increase in organic traffic within the first quarter of implementation
- 30% growth in online sales post-Knowledge Graph integration
- 50% of searches resulting in amplified visibility through zero-click results
Companies leveraging specialized tools and expert guidance from agencies like JEMSU and WordLift have experienced significant competitive advantages.
Through AI-driven semantic annotation and structured data optimization, businesses have secured prominent positions in Featured Snippets and Knowledge Panels, establishing greater brand authority.
This technical approach to SEO has proven particularly effective for specialized retailers seeking niche market dominance.
Graph Implementation Results
Examining detailed case studies of Knowledge Graph implementation reveals convincing performance metrics across diverse industries.
Structured data integration has demonstrated significant improvements in search visibility, with organizations reporting up to 35% increases in click-through rates and 20% gains in organic traffic following Knowledge Graph optimization.
Implementation analysis shows that organizations leveraging schema markup and JSON-LD achieve amplified search engine understanding, resulting in a 30% propel in organic traffic and 45% improvement in first-page rankings for key products. The use of structured data markup tools like Google's Structured Data Testing Tool ensures proper validation and implementation.
The strategic use of structured data has proven particularly effective in generating rich snippets, knowledge panels, and augmented search descriptions, leading to measurable improvements in user engagement metrics.
Technical implementation data indicates that successful Knowledge Graph integration relies heavily on semantic search optimization and natural language processing capabilities.
Organizations implementing holistic data organization strategies, coupled with structured markup, consistently achieve superior visibility in search results.
The integration of APIs for adaptive content updates, combined with resilient data governance architectures, has shown to be instrumental in maintaining sustained Knowledge Graph presence and improved search engine visibility across multiple platforms.
SEO Performance Breakthroughs
Convincing case studies across diverse industries showcase extraordinary SEO performance breakthroughs achieved through Knowledge Graph implementation.
Organizations leveraging entity-based content models and structured data have documented significant increases in organic traffic, keyword rankings, and SERP visibility. These implementations demonstrate how strategic content organization, coupled with Knowledge Graph design, drives sustainable SEO performance improvements.
Analysis of successful implementations reveals consistent patterns of achievement:
- Entity-based content modeling resulted in 30-50% improvements in search visibility, particularly for complex topics requiring deep situational understanding
- Implementation of schema markup and structured data led to rich result acquisitions, increasing SERP real estate and click-through rates
- Knowledge Graph integration enabled more effective keyword targeting and expansion, leading to substantial growth in organic keyword portfolios
- Strategic content restructuring and optimization yielded measurable increases in goal completions and conversions
Technical SEO challenges, including content cannibalization and scalability issues, were effectively addressed through Knowledge Graph implementation.
Organizations reported amplified user experiences, improved local search performance, and sustained organic traffic growth. The data consistently demonstrates that Knowledge Graph adoption, combined with strategic content optimization, delivers measurable SEO performance breakthroughs across multiple metrics.
Frequently Asked Questions
How Long Does It Typically Take to Get Included in Google's Knowledge Graph?
Incorporation in Google's Knowledge Graph typically takes 2-6 months, contingent upon entity recognition, data quality, and verification processes. Regular algorithmic updates occur every 2-3 weeks, influencing integration timelines.
Can Small Businesses Effectively Compete With Larger Brands Using Knowledge Graphs?
Small businesses can effectively compete with larger brands through focused knowledge graph optimization, quality content creation, accurate local data, and strategic PR efforts, despite having fewer resources than major competitors.
What Are the Costs Associated With Implementing Knowledge Graph Optimization?
Implementation costs for knowledge graphs range from $50,000-$100,000 for basic POCs, while full-scale projects cost $1-3 million. Monthly infrastructure costs vary between $338-$2,000, depending on chosen platform and requirements.
Do Knowledge Graphs Impact Local SEO Differently Than National or Global SEO?
Knowledge Graphs have a more concentrated impact on local SEO, focusing on geographic-specific information and hyperlocal visibility, while national/global SEO employs broader data patterns and competition metrics across wider market segments.
How Often Should Knowledge Graph Data Be Updated for Optimal SEO Performance?
For optimal performance, update knowledge graph data every 2-3 weeks to align with Google's update frequency. Critical business changes should be implemented immediately, while routine updates should follow Google's major update cycles in July/December.
Conclusion
FINALE:
Knowledge graphs represent a critical advancement in search engine optimization, integrating semantic relationships and structured data to amplify search relevance and user experience. The implementation of knowledge graph technologies demonstrates measurable improvements in SERP visibility, click-through rates, and user engagement metrics. As machine learning algorithms evolve, the fusion of knowledge graphs with SEO practices will continue driving more intelligent search responses and precise entity recognition, conclusively shaping the future of digital information retrieval and visibility optimization.