Natural Language Processing (NLP) significantly improves SEO by refining search engines' abilities to comprehend user intent and the setting of queries. Advanced models like BERT enhance the interpretation of complex language, shifting the focus from simple keyword matches to understanding layered meanings. This leads to more precise, intent-driven content alignment, promoting engagement. Semantic search technologies and entity recognition further refine content according to real user needs. As a result, businesses see improved search rankings and user satisfaction. By exploring further, revelations into these innovations can guide superior strategy development in the rapidly changing online environment.
Learn More
- NLP enhances SEO by improving keyword interpretation and understanding user intent in search queries.
- BERT's architecture excels in understanding complex language, improving relevance of search results through contextual analysis.
- Semantic search shifts optimization from keywords to user intent, aligning content with search intent for better SEO performance.
- Voice search optimization targets natural dialogue patterns, enhancing SEO with question-based, long-tail keywords.
- Advanced NLP techniques help businesses maintain competitive SEO rankings by adapting to evolving user needs and search algorithms.
NLP's Role in Search
NLP's role in search is revolutionary, reshaping how search engines interpret and respond to user queries. With the integration of Natural Language Processing, search engines now excel at situational analysis by examining how keywords are embedded within the broader content. This advancement allows search engines to understand the intricate relationships between words, thereby improving the relevance and precision of search results. Semantic search is a crucial byproduct, enabling engines to discern situation and meaning beyond basic keyword matching. Moreover, NLP improves keyword relevance by evaluating the situational importance of terms, thereby elevating search result quality. The emphasis on content quality over keyword density is significant, as NLP-driven algorithms prioritize important information over simple keyword repetitions. This necessitates a shift in SEO strategies, as websites must adjust to these changing algorithms to maintain or improve rankings. Furthermore, NLP's flexibility extends beyond content, incorporating deep learning models that enhance the understanding of natural language queries. Search engines use algorithms that recognize natural language phrases and entities for indexing, which is crucial for indexing and retrieving relevant content. Algorithms like BERT improve search result relevance and accuracy by processing words in relation to all other words in a sentence. These large language models (LLMs) utilize vast datasets and computational power to enhance language understanding capabilities. This progression encourages an ongoing process where past searches inform future situations. NLP's capacity for cross-medium search further highlights its revolutionary influence, allowing extensive searches across texts, videos, and images.
Understanding User Intent
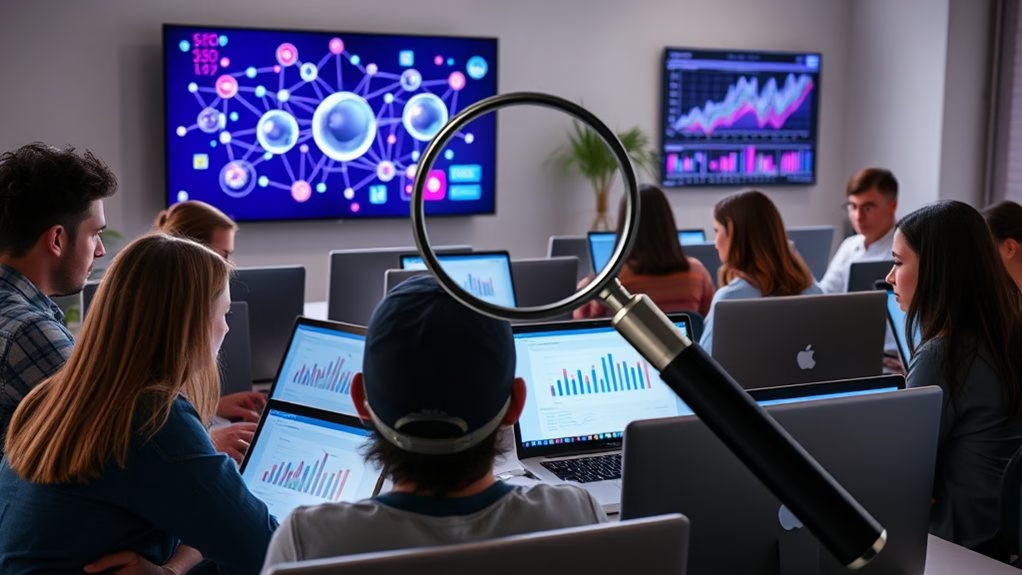
Understanding user intent is crucial for optimizing search engines to deliver intent-driven results that improve user satisfaction. By employing Natural Language Processing (NLP), search engines can more accurately decipher query surroundings, allowing algorithms to interpret the subtleties of user queries and provide relevant results that align with the searcher's purpose. This semantic user interaction not only enhances the relevance of search outcomes but also strategically positions websites to meet user needs, thus boosting SEO performance and driving engagement. Understanding search intent is essential as it influences content performance in search rankings, with Google's algorithm prioritizing content that matches user intent over mere keywords. Aligning content with user intent enhances the search experience and reduces bounce rates, ensuring that users find what they are looking for more efficiently. User intent can be classified into four main types, which helps in developing content that effectively addresses varying user needs.
Deciphering Query Context
Deciphering query circumstances is vital in understanding user intent, a key component in improving search engine optimization strategies. NLP technologies, such as Google's BERT model, utilize advanced algorithms to interpret ambiguous language, allowing for a thorough grasp of the situation within search queries. By analyzing the entire sentence, NLP can discern whether a search is informational, navigational, or transactional, enabling content creators to tailor their material to meet specific user needs effectively. Furthermore, NLP enhances online content visibility and effectiveness, which aids in improving search visibility and driving organic traffic to websites. Google's BERT model enhances the understanding of ambiguous language by analyzing the context of entire sentences, which improves the topical relevance of search results.
Semantic analysis, supported by NLP, improves the capability to interpret the intended meaning of words beyond mere keyword matches. This allows search engines to differentiate between different meanings of the same word, ensuring that the results align with the user's true intent. The emphasis on semantic search also highlights the importance of optimizing for long-tail keywords, which are essential for capturing informal subtleties in natural language. Additionally, the integration of structured data markup provides context to search engines, further enhancing content comprehension and visibility on search engine results pages.
Moreover, NLP's situational understanding enables search engines to perform sentiment analysis, recognizing the emotional tone behind queries. This intricate comprehension aids in distinguishing subtle differences in meaning based on additional words used in a query, ensuring that search results are not only relevant but also aligned with the user's underlying purpose.
Intent-Driven Search Results
Utilizing the capabilities of Natural Language Processing (NLP) in search engine optimization is essential for producing intent-driven search results that align closely with user expectations. NLP algorithms have transformed SEO by enabling the identification of user intent, which can be informational, transactional, directional, or commercial investigation in nature. This advancement allows for a more intricate understanding of search queries beyond mere keyword matching, ensuring that users receive content that truly meets their needs.
- Intent Identification: NLP tools analyze search queries to discern underlying intentions, altering how content is customized to meet user demands. By recognizing whether a query seeks information, desires a transaction, or directs to a specific site, search engines can deliver more relevant results. The focus on user intent rather than strict keyword matching refines SEO methodologies, aligning content with the diverse expressions of user queries.
- Contextual Analysis: By examining the semantics and backdrop of queries, NLP improves the accuracy and relevance of search results. This strategic depth allows SEO practitioners to create content that connects with user expectations, leading to higher engagement and conversion rates. Understanding user intent guides content relevance and optimization, ensuring users find precisely what they are looking for.
- Keyword Clustering: Through semantic keyword clustering, NLP aids in organizing content around user-centric themes. This strategic approach not only boosts search visibility but also aligns content with the diverse queries users may employ, ensuring thorough coverage of user interests.
Semantic User Interaction
In the developing environment of search engine optimization, semantic user interaction stands as an essential strategy for improving user intent comprehension. Semantic search enhances search engines' ability to comprehend user queries by focusing on relationships between concepts. By leveraging situational signals such as user location, past searches, and preferences, search engines can tailor results to meet specific user needs. This approach transcends mere keyword matching, instead utilizing advanced natural language processing (NLP) and natural language understanding (NLU) tools to decode and expand user queries. Machine learning models play a crucial role in semantic search by analyzing vast amounts of data to identify patterns, thus enabling search engines to anticipate user needs more effectively. By utilizing vector embeddings to map meanings of words and phrases, semantic search aligns with user intent and enhances the overall search experience.
Aspect | Approach | Benefit |
---|---|---|
Situational Signals | Incorporate user data and location | Customized search results |
Entity Recognition | Identify specific entities like people or places | Increased result accuracy |
Conceptual Meanings | Match queries with underlying intent | Improved semantic relevance |
Vector Similarity | Calculate similarity between query and document vectors | Enhanced content matching |
Machine Learning | Use models like SBERT for semantic relevance | Better user intent decoding |
Strategically, semantic processing involves employing AI models for semantic relevance and query expansion through synonyms and typo tolerance. This guarantees a more intuitive user experience by aligning search results with the user's actual intent. By focusing on entity-based optimization and situational relevance, businesses can improve content visibility, thus increasing user engagement and staying ahead of algorithm updates. This all-encompassing approach not only improves search relevance but also fortifies SEO resilience.
Semantic Search Impact
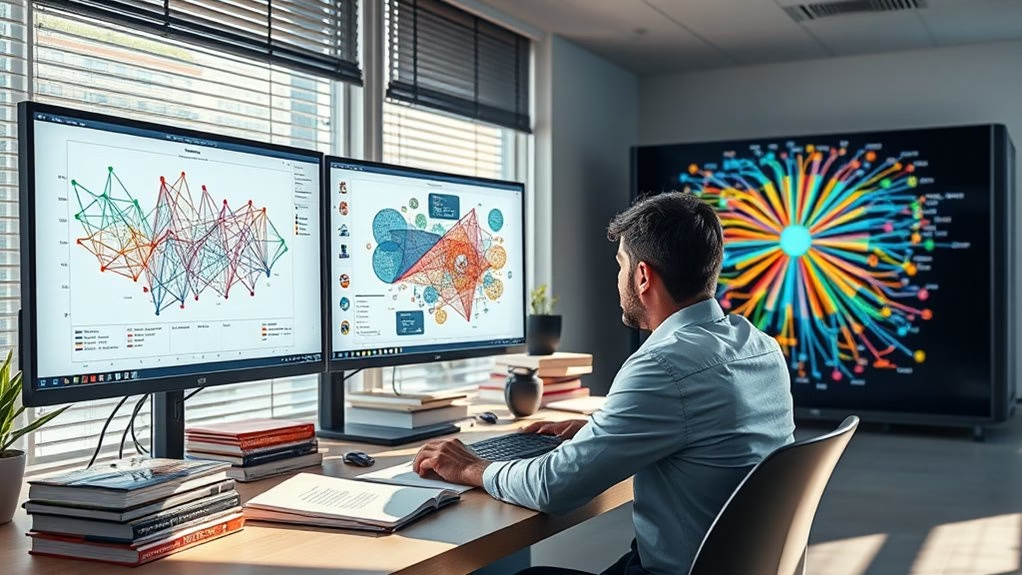
Amidst the developing environment of search technologies, semantic search has emerged as a revolutionary force impacting SEO strategies significantly. This transformation is driven by the ability of semantic search to interpret user intent and situational meanings, facilitated by Natural Language Processing (NLP) and Machine Learning (ML). Consequently, SEO strategies are advancing from a keyword-centric focus to a more comprehensive approach centered around topics. This transition is underpinned by several key observations:
- Shift from Keywords to Topics: In optimizing for semantic search, emphasis is placed on generating extensive content that addresses user intent. This alignment with user needs not only improves SERP rankings but also boosts user satisfaction.
- Entity Recognition and Knowledge Graphs: Utilizing entity recognition and knowledge graphs improves the ability to understand and connect various entities, providing richer and more relevant search results. Knowledge graphs, which enhance semantic searches by representing relationships between different web entities, offer insights into customer intent and help businesses understand user preferences and behaviors. Websites also integrate tools like reCAPTCHA to protect against bot traffic and ensure genuine user interactions.
- Structured Data Markup: Implementing structured data markup, such as Schema, is essential in helping search engines comprehend content relevance, thereby improving visibility and ranking. Semantic search enhances search results by focusing on user intent, which leads to targeted and meaningful results.
BERT and Language Models
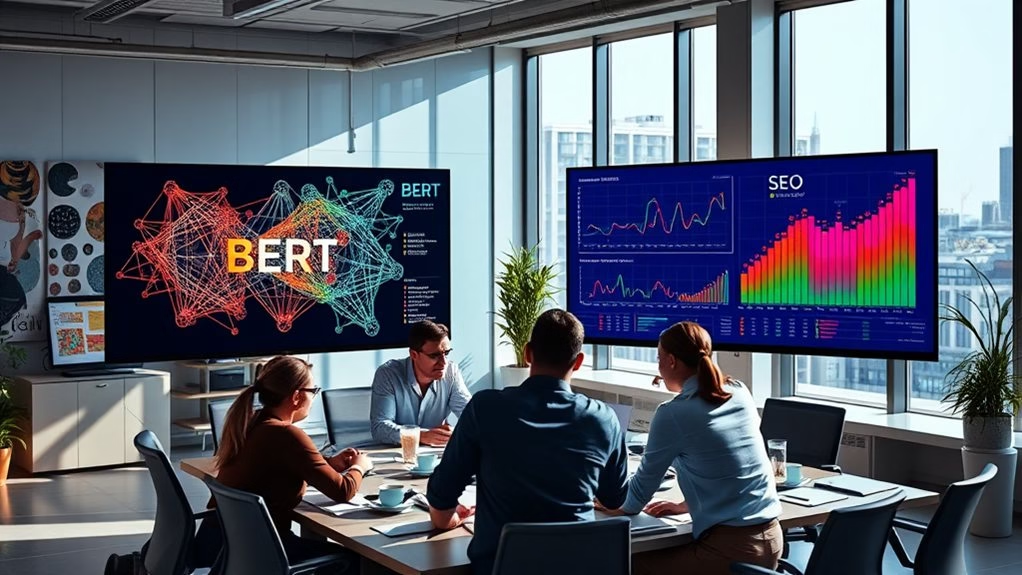
BERT's introduction has transformed query processing by enabling deeper situational understanding, significantly enhancing search engines' ability to interpret complex language patterns. As a critical advancement in language models, BERT's bidirectional training method allows it to grasp subtle meanings and relationships within text, outperforming previous unidirectional models. This leap in understanding not only improves search results but also sets a new standard for language models, inspiring further innovations that continue to enhance SEO strategies across diverse linguistic environments. BERT utilizes the self-attention mechanism to process entire sentences at once, allowing it to capture comprehensive contextual word relationships, which is a foundational aspect of its transformational impact on natural language processing. By utilizing the Transformer encoder architecture, BERT processes entire word sequences simultaneously, which contributes to its superior performance in understanding complex language tasks. The WordPiece tokenizer used by BERT is essential in converting text to tokens, ensuring that even rare words can be effectively represented and understood within the model's framework.
BERT's Impact on Queries
Taking a step forward in the realm of search engine optimization, BERT's impact on queries marks a significant shift towards understanding situational subtleties in user searches. By employing situational analysis, BERT transforms how search engines interpret queries, enhancing relevance and precision.
One of its primary advances is its ability to process prepositions and conjunctions, which often alter the meaning of queries drastically. This capability allows BERT to discern keyword flexibility, moving beyond exact matches to grasp broader situational meanings. BERT's training on a massive corpus like the Wikipedia plain text corpus enables it to develop a deep understanding of language context, which is crucial for identifying nuanced meanings in search queries. BERT's bidirectional contextual word understanding allows it to interpret language in a manner similar to human comprehension, making it more advanced than previous algorithms.
The implications of BERT's functionality are significant, particularly in its impact on search results:
- Enhanced Search Accuracy: BERT influences approximately 10% of English searches in the U.S., providing more situationally accurate results without penalizing existing web content. It achieves this by improving matching of queries with relevant results, ensuring that users find the information they seek more efficiently.
- Long-Tail Keyword Importance: As BERT emphasizes understanding intent, long-tail keywords become increasingly essential for capturing subtle user intent.
- Improvement in Featured Snippets: By focusing on query situation, BERT guarantees featured snippets are more relevant to user searches.
Strategically, BERT encourages a shift from keyword-centric strategies toward creating high-quality, situationally rich content. Its bidirectional analysis enhances query understanding, guaranteeing that SEO efforts align with the advancing terrain of search engine technology.
Language Model Advancements
In recent years, advancements in language models have redefined the capabilities of natural language processing (NLP) technologies, with BERT and its successors leading the charge. BERT, or Bidirectional Encoder Representations from Transformers, has set a new standard in understanding word surroundings by employing transformer designs. Pre-trained on tasks like Masked Language Model (MLM) and Next Sentence Prediction (NSP), BERT's bidirectional approach allows for a deeper comprehension of language subtleties, significantly outperforming earlier models. With two variants, BERT BASE and BERT LARGE, it has become a benchmark in NLP experiments, particularly excelling in sentiment analysis and natural language understanding (NLU). Building on BERT's foundation, RoBERTa improves performance by refining the training process and focusing solely on MLM with shifting masking patterns. By leveraging a byte pair encoding (BPE) system with a larger vocabulary, RoBERTa often surpasses BERT in benchmark tests, though it demands more computational resources. Trained on larger datasets and incorporating dynamic masking, RoBERTa's robust architecture enhances its adaptability and effectiveness in various NLP tasks. BERT's architecture is defined by two parameters: L (number of layers) and H (hidden size), which determine its depth and capacity for understanding complex language structures. The use of transformer encoder mechanisms allows BERT to learn contextual relations between words and sub-words, improving its overall language comprehension. These models have been crucial in improving search engine results, language translation, and interactive AI. As we anticipate future trends, ongoing efforts intend to improve BERT's situational understanding while reducing resource requirements, promising a more accessible and sophisticated suite of NLP tools for diverse applications.
Understanding Complex Language
Understanding complex language through advanced language models has altered natural language processing, led by frameworks like BERT. This model, with its bidirectional training and transformer encoder, excels in understanding the setting of words within sentences.
BERT's design is pre-trained on vast amounts of unlabeled data, utilizing techniques such as the Masked Language Model (MLM) and Next Sentence Prediction (NSP) to improve its situational comprehension. This enables BERT to perform state-of-the-art tasks such as Named Entity Recognition (NER), question-answering, and content classification, thereby raising the performance of NLP applications. BERT's architectural family is defined by two parameters: L (number of layers) and H (hidden size), with notable examples like BERT BASE (12L/768H) and BERT LARGE (24L/1024H) showcasing its transformer architecture efficiency. Furthermore, BERT's open-source nature allows organizations to fine-tune the model for specific applications, enhancing its versatility across different domains.
BERT's impact extends significantly into search engine optimization. Here are three critical effects:
- Improved Query Understanding: By interpreting search queries more accurately, BERT enhances the relevance of search results.
- Multilingual Capabilities: Supporting over 70 languages, it elevates the accuracy of voice searches and cross-language information retrieval.
- AI Model Inspiration: It has inspired lighter versions and other models, such as GPT-2, which further polish NLP capabilities.
Despite its achievements, BERT's encoder-only design limits its content generation ability, and its computational demands are substantial.
However, its strategic influence on SEO and NLP continues to be significant, driving advancements in situational language understanding.
Entity Recognition Techniques
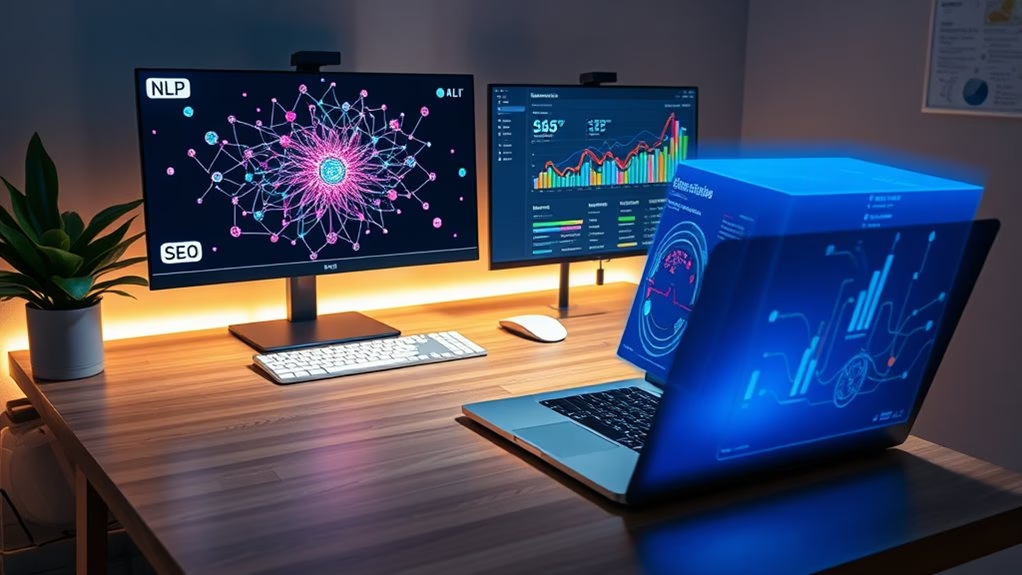
Entity recognition techniques are essential in the domain of Natural Language Processing (NLP) as they enable the precise extraction of meaningful entities from vast text datasets. These techniques range from rule-based to machine learning and deep learning methods, each contributing distinct strengths to the table.
Rule-based methods employ predefined pattern-based and situational rules for identifying entities such as dates and email addresses. Though effective for specific formats, they demand expert knowledge and become complex with varied text structures.
In contrast, machine learning-based methods utilize statistical models, like Support Vector Machines and conditional random fields, to detect entities, even amidst spelling variations. These require substantial training data, but they excel in recognizing entities like product names and prices.
Deep learning techniques, such as those utilizing BERT and transformers, further improve entity recognition by understanding semantic and syntactic relationships within text. They can be fine-tuned for specific datasets, making them highly effective for large and diverse datasets with minimal human input.
The strategic implementation of these techniques involves careful data acquisition, model fine-tuning, and algorithm selection, ensuring improved accuracy. This versatility finds applications across domains, from healthcare to finance, underscoring their indispensable role in NLP and SEO.
Contextual Relevance in SEO
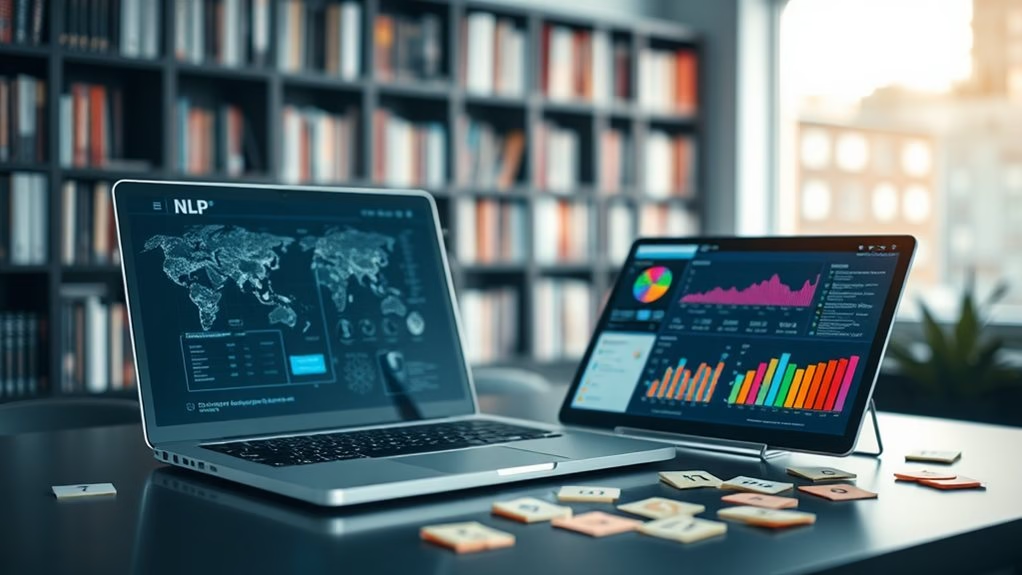
The integration of advanced entity recognition techniques within Natural Language Processing (NLP) sets a strong foundation for improving situational relevance in SEO.
Situational relevance, defined as the strategic alignment of content with the topic or keyword, is essential for SEO success. It enables search engines like Google to effectively comprehend the relevance and quality of web pages, thus improving their ranking and authority.
Relevant links are instrumental in this process, as they guide users to pertinent sections, augmenting the user experience and improving content quality.
Incorporating situational relevance into an SEO strategy involves several key practices:
- Internal Linking: Developing an effective internal linking strategy increases a site's authority by clearly illustrating its structure to search engine algorithms. This approach not only improves rankings but also enhances user guidance.
- Strategic Placement: Placing relevant links in significant sections of a website guarantees users are directed to relevant information, nurturing user engagement and reducing bounce rates.
- High-Quality Sources: Securing links from authoritative websites with substantial Domain Authority (DA) or Domain Rating (DR) is vital. It strengthens domain authority and demonstrates the website's relevance within its industry or niche.
Voice Search Optimization
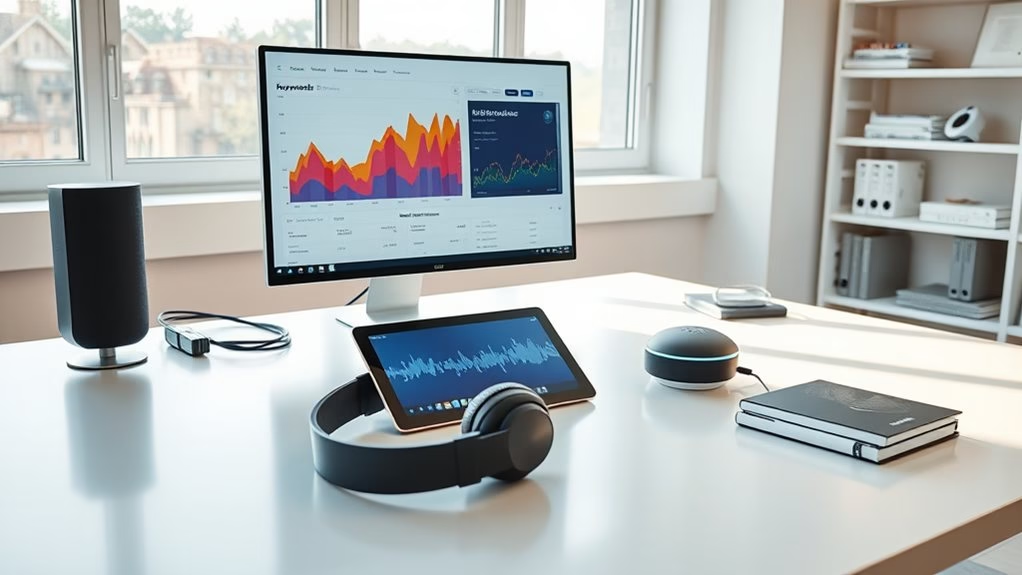
With the rapid development of technology, refining for voice search has become an essential element in contemporary SEO strategies. As voice-activated devices proliferate, businesses must adjust by targeting question keywords and employing schema markup to capture featured snippets. The strategy involves using long-tail keywords that reflect natural dialogue patterns, guaranteeing content is structured with headers and lists, and embedding these keywords as answers to frequently asked queries.
Strategy Component | Key Actions | Benefits |
---|---|---|
Target Question Keywords | Use question-based, long-tail keywords | Improves natural language match |
Technical Optimization | Confirm mobile compatibility and page speed | Enhances user experience |
Featured Snippets | Apply structured data and concise answers | Increases visibility |
Local SEO Focus | Refine for local queries and update business listings | Amplifies local search relevance |
Technical considerations such as refining for mobile and speed are paramount, as they directly influence ranking and user satisfaction. Responsive design and HTTPS security are essential for maintaining data integrity and efficiency. In addition, improving local SEO through updated Google My Business listings and employing location-specific content can significantly enhance the chances of appearing in "near me" voice searches. By strategically aligning these components, businesses can stay competitive in the changing environment of voice search optimization.
Content Quality and NLP
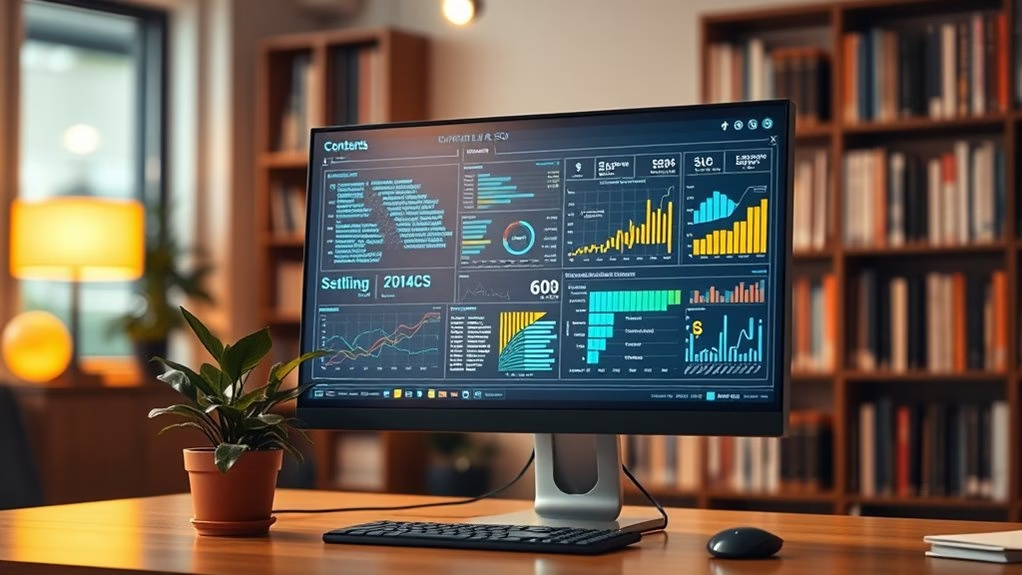
A significant advancement in the field of SEO is the integration of Natural Language Processing (NLP) to improve content quality, thereby aligning it with user intent.
NLP plays a fundamental role in altering content optimization strategies by focusing on user needs rather than traditional keyword-centric approaches. By understanding the framework of search queries, NLP guarantees that content is not only relevant but also satisfies the underlying intent, thus improving user experience.
This strategic shift is evidenced through several key techniques:
- Entity Recognition: NLP employs entity recognition to discern the framework and importance of content elements, aiding search engines in more accurate content ranking. This leads to improved visibility for high-quality content that addresses user queries effectively.
- Semantic Analysis: Through semantic analysis, NLP deciphers the meaning and intent behind content, guaranteeing it aligns with user queries. This results in content that is not only relevant to the framework but also boosts search engine rankings.
- BERT Integration: By integrating with BERT, NLP can analyze entire sentences rather than isolated keywords, nurturing more natural and meaningful content creation that connects with users.
Ultimately, NLP's capability to improve content quality through these advanced techniques positions it as a significant component in modern SEO strategies.
Future of NLP in SEO
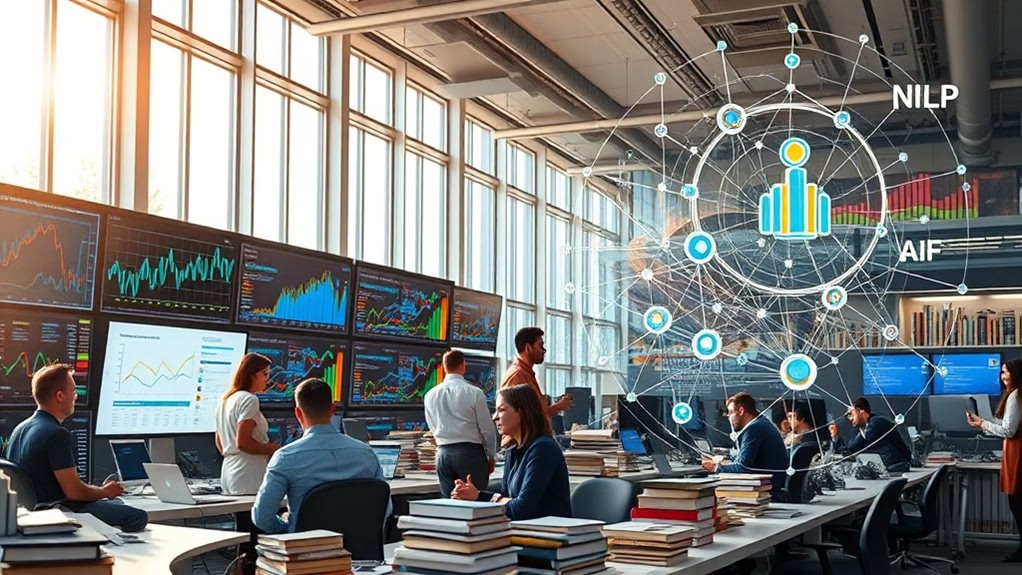
Anticipation surrounds the future role of Natural Language Processing (NLP) in shaping SEO strategies, as it promises to revolutionize how digital content is improved and accessed. NLP's evolution emphasizes understanding user intent and situation over mere keyword matching, significantly impacting search engine optimization.
By focusing on semantic search, NLP can connect user queries with content based on meaning, not just isolated keywords, thereby fine-tuning search accuracy and relevance.
The integration of NLP with AI-generated content is ready to advance this evolution, offering more precise search results. As voice and multimodal searches gain traction, content must adjust to informal keywords, requiring a shift towards natural language enhancement. This shift promotes high-quality, intent-driven content that meets user needs, moving away from traditional keyword density models.
NLP's ability to analyze content sentiment, situation, and readability will further improve SEO strategies, aligning them with AI-driven perspectives. The strategic adjustment of SEO to these trends guarantees competitive advantage.
Frequently Asked Questions
How Does NLP Affect Local SEO Strategies?
Understanding local language subtleties significantly improves the precision of local search strategies. By focusing on user intent and situation, businesses can refine their content, boosting visibility, engagement, and authority in local search results.
What Role Does NLP Play in E-Commerce SEO?
In e-commerce, NLP enhances content by understanding user intent, improving product descriptions, and personalizing customer interactions. This improves search rankings, increases user engagement, and drives conversion rates, proving its essential role in strategic digital marketing.
Can NLP Improve Video Content SEO?
Yes, through semantic understanding and search intent analysis, video content can achieve improved visibility and user engagement. This strategic optimization promotes better click-through rates and flexibility to changing AI-driven search algorithms, ultimately increasing SEO performance.
How Does NLP Influence SEO for Multilingual Websites?
NLP significantly improves multilingual website SEO by accurately interpreting user intent and situation across languages, improving content localization. AI models like BERT and MUM aid in ranking, while sentiment analysis guarantees culturally resonant and engaging user experiences.
What Tools Utilize NLP for SEO Analysis?
Numerous tools integrate advanced language processing for digital analysis, including SEOptimer and Scalenut for keyword optimization, Google NLP API for text analysis, and Ryte Content Editor for improving content relevance and readability, enhancing digital visibility and engagement.
Conclusion
The integration of natural language processing (NLP) with search engine optimization (SEO) signifies a revolutionary shift in digital strategies. By enhancing the understanding of user intent through semantic search and leveraging advanced models like BERT, SEO practices are increasingly improved. Entity recognition and situational relevance further strengthen content optimization, while the rise of voice search highlights the necessity for flexible techniques. As NLP continues to evolve, its impact on SEO will drive more precise, user-centric digital experiences, emphasizing its strategic importance in future developments.